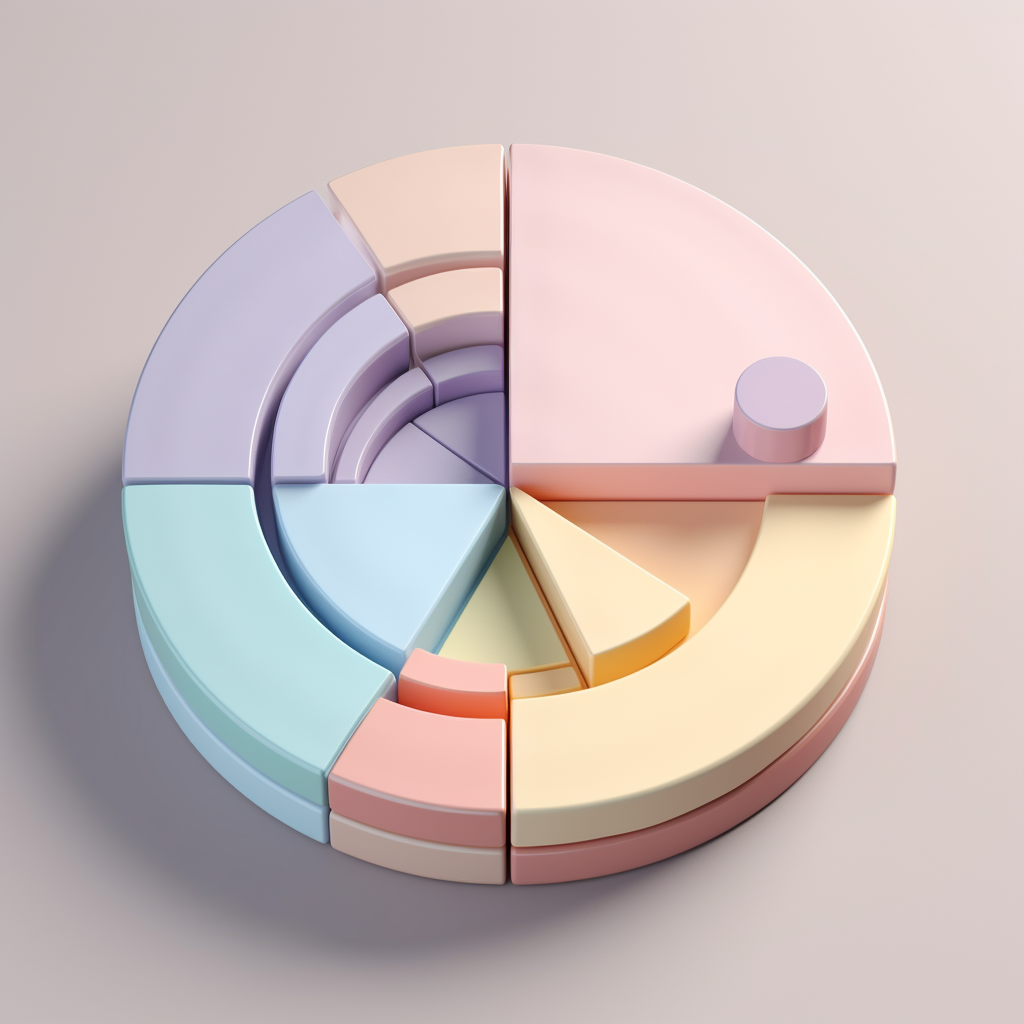
The Science Behind Credit Scoring in the Digital Age
Credit scoring is the process of evaluating a borrower's creditworthiness based on various factors such as income, payment history, debt level, and credit mix. Lenders, including banks, credit card companies, and online platforms like embedded lending, use credit scoring to determine whether to extend credit, how much credit to offer, and at what interest rate.
Traditionally, credit scoring has relied on data from credit bureaus, which collect information from lenders and other sources regarding consumers' credit history and behavior. One of the most widely used credit score ratings is FICO, which calculates credit scores based on payment history, amounts owed, length of credit history, new credit, and types of credit used, as shown in the chart below:
Source: https://www.cpapracticeadvisor.com/2014/03/11/fico-to-release-new-credit-scoring-system/14595/
However, a drawback of this scoring system is that if you don't frequently use banks, your score may be low because you may lack credit history, new credit, or payment history. Approximately 22% of Americans either lack sufficient credit history or have none at all, making it difficult to calculate a score.
Credit scoring is currently undergoing a transformation due to the advent of big data, which refers to the large volume, variety, velocity, and veracity of data generated from various sources, including social media, e-commerce, mobile devices, sensors, and satellites. Big data can provide new insights into consumer behavior, preferences, and needs, which can be harnessed to enhance products and services.
In the context of credit scoring, big data offers several advantages for both lenders and consumers:
- Expanding Access to Credit: Big data enables lenders to reach underserved populations lacking traditional credit history or with low credit scores. By using alternative data sources such as digital footprints (data generated when people access or register on websites), lenders can assess the creditworthiness of borrowers who are otherwise unscorable or invisible to the credit system. For example, a study by Berg et al. (2020) found that even simple variables from the digital footprint, such as the device type or email provider used by the consumer, can equal or exceed the information content of credit bureau scores.
- Improving Accuracy and Efficiency: Big data allows lenders to enhance the accuracy and efficiency of credit scoring models by incorporating more relevant and timely data that capture the dynamic and complex nature of consumer behavior. Advanced analytical techniques such as machine learning and artificial intelligence enable lenders to process large amounts of data faster and more accurately than traditional methods. For example, a review by Onay and Öztürk (2018) found that machine learning methods can outperform conventional statistical methods regarding predictive performance and computational speed.
- Enhancing Customer Experience: Big data empowers lenders to offer more personalized and convenient services by leveraging data-driven insights. Lenders can tailor their products and offers to meet the specific needs and preferences of each customer. They can also streamline their processes and reduce costs by automating tasks such as data collection, verification, and decision-making.
A study conducted by the Journal of Empirical Finance demonstrated that credit scoring calculated using big data is more accurate than traditional credit scoring methods. The charts below illustrate the relationship between credit scoring (the blue bars) and overdue percentage (the red line). In the case of big data credit scores, there is a more precise correlation: as the score increases, the overdue percentage decreases, compared to traditional scoring shown in the second chart.
Source: https://ars.els-cdn.com/content/image/1-s2.0-S0927539821000098-gr1.jpg
However, big data also presents challenges and risks for both lenders and consumers:
- Ensuring Data Quality: Big data may suffer from issues such as incompleteness, inconsistency, noise, bias, and fraud that can affect the quality and reliability of the data. Lenders must ensure that they use accurate and relevant data sources that are verified and validated for their intended purposes. They should also regularly monitor and update their data to reflect changes in consumer behavior and market conditions.
- Protecting Data Privacy: Big data raises concerns about the privacy and security of the data collected and used by lenders. Consumers may not be aware of how their data are collected, shared, or used by third parties, and they may lack control or consent over their data's use. Therefore, lenders must respect consumers' privacy rights and comply with applicable data protection laws and regulations. They should also implement measures to safeguard their data from unauthorized access or misuse.
- Preventing Data Discrimination: Big data introduces the potential for discrimination or unfairness in credit scoring decisions. Consumers may be excluded or disadvantaged based on characteristics or behaviors not directly related to their creditworthiness. For instance, some variables from the digital footprint may inadvertently reflect a consumer's gender, race, ethnicity, religion, or political affiliation. Therefore, lenders must use transparent and explainable methods that adhere to ethical principles and legal norms of nondiscrimination and equal opportunity.
Credit scoring is a crucial tool for promoting financial inclusion and economic development. In the age of big data, credit scoring is evolving rapidly to leverage new data sources and analysis methods that can enhance the quality and efficiency of credit decisions. However, big data also introduces new challenges and risks that necessitate careful consideration and regulation to protect consumers' rights and promote social welfare.
Credit scoring, financial inclusion, big data credit scoring, credit decision analytics, advanced credit scoring methods, credit scoring data sources, credit scoring evolution, consumer credit analysis, credit risk assessment, big data in finance, financial data analysis, credit scoring challenges, credit scoring regulations, consumer rights in lending, social welfare and credit scoring, credit decision efficiency, credit scoring and AI